News • 1/26/2024
Continuous benchmarking of algorithms accelerates development of audio diagnostic for Tuberculosis
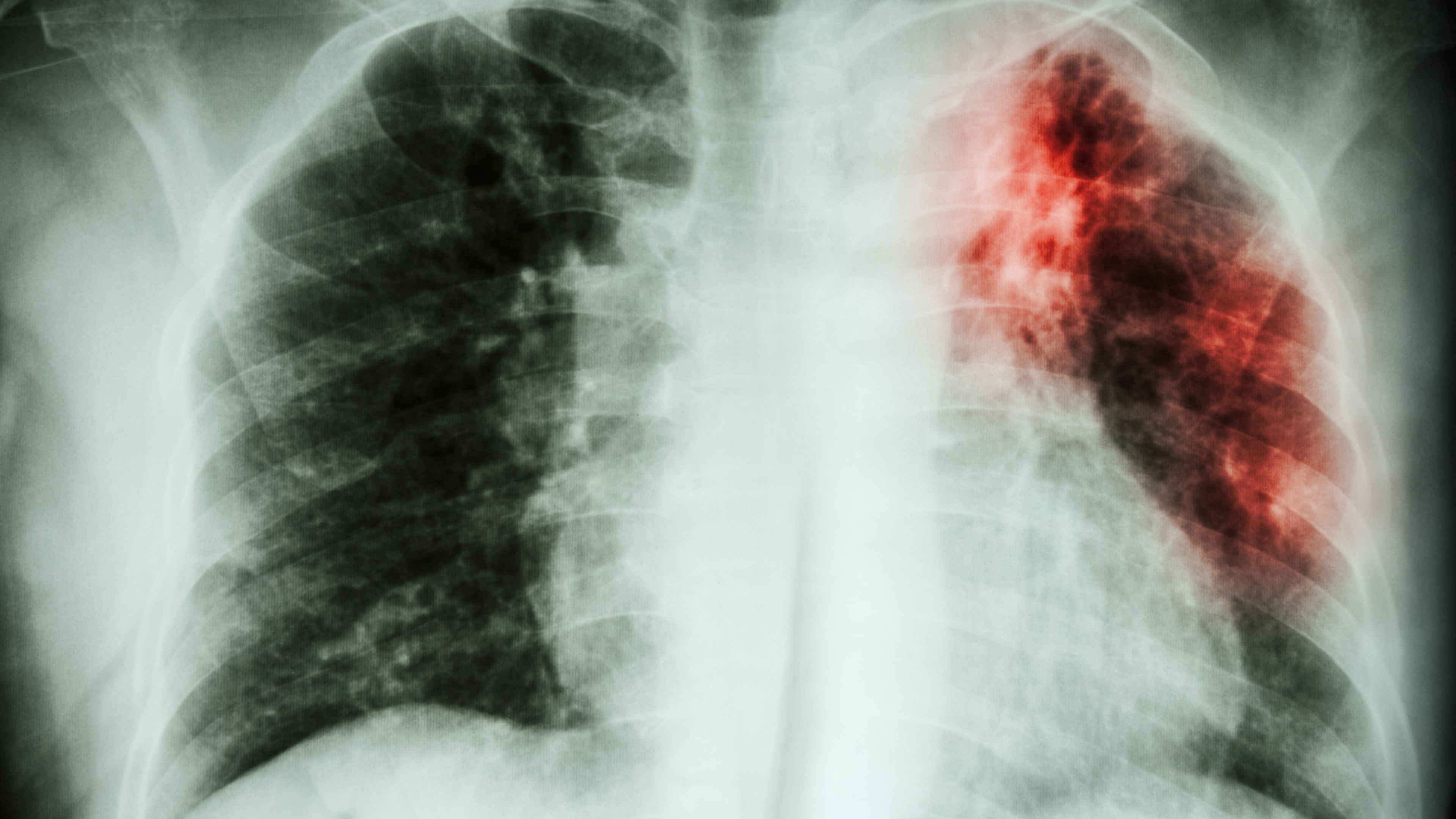
The CODA TB DREAM Challenge invites registrants to submit new machine learning models that can be evaluated for their predictive power.
SEATTLE, WA – The COugh Diagnostic Algorithm for Tuberculosis (CODA TB) DREAM Challenge has entered into its continuous benchmarking stage, enabling ongoing submission of new algorithms that can predict the presence of Tuberculosis (TB) from cough recordings. This stage allows registrants to evaluate models for generalizable performance, advancing the development of reliable technologies that could be deployed in a clinical setting.
“Challenges represent a valuable mechanism to engage researchers to work on important problems in the predictive modeling space,” says Solveig Sieberts, PhD, Director of Digital Health at Sage Bionetworks. “We are excited to provide this continuous benchmarking resource for researchers to build upon what we learned in the CODA TB challenge and to invite new researchers to get involved.”
Co-organized by Sage Bionetworks, University of Montreal, University of California San Francisco and Global Health Labs, the CODA TB DREAM Challenge invited community computational researchers to predict TB status using the audible features from elicited coughs collected through a smartphone app. Researchers generated predictive models using the cough recordings alone, or in combination with standard demographic and clinical screening factors. Predictive models were trained on diverse data, which were collected from people in 7 countries across Africa and Asia who presented to clinics with new or worsening cough for at least 2 weeks.
Following the announcement of the winning submissions in March 2023, the challenge recently entered its state of continuous benchmarking, allowing open submission of new models for unbiased evaluation and benchmarking against a common standard. The continuous benchmarking evaluations leverage an expanded test dataset (“held-out”) beyond that used during the initial challenge. This will provide researchers with an independent evaluation of a model’s generalizable performance—a crucial indicator of how well the model is expected to perform on new, unseen data for real-world applications.
“Together with the availability of the CODA TB training dataset, this represents a valuable resource to enable researchers to develop and assess diagnostic models for TB, ultimately speeding up the development of clinically-relevant technologies,” says Adithya Cattamanchi, MD, Professor of Medicine at University of California San Francisco.
As cough is a common symptom of TB, scientists believe that it has the potential to be used as a key biomarker for disease diagnosis—a growing field known as “acoustic epidemiology.” Indeed, several studies have shown that cough sounds may be used to screen for TB. Public challenges that build and refine AI-based cough tools now have the ability to improve the predictive power of these technologies and accelerate their uptake into the clinic.
Tuberculosis is a communicable, bacterial infection caused by Mycobacterium tuberculosis, which affects over 10 million people worldwide each year. Despite being a preventable and curable disease, 1.5 million people die from TB every year, making it the world’s second leading cause of death from infectious disease, after COVID-19.
Due to failures in testing or significant challenges in accessing health facilities, approximately 40% of people who contract TB are not diagnosed or reported to public health authorities. Researchers hope that the development of low-cost, non-invasive digital screening tools may improve some of the gaps in diagnosis and reduce the global burden of disease.
Latest News
No articles